A5
.docx
keyboard_arrow_up
School
University of Texas, Dallas *
*We aren’t endorsed by this school
Course
4352
Subject
Management
Date
May 5, 2024
Type
docx
Pages
3
Uploaded by AmbassadorMonkeyPerson711 on coursehero.com
Assignment 5
Khushi Balar (kxb210017)
Jindal School of Management, The University of Texas at Dallas
ITSS 4352 : Web Analytics
Professor. Vatsal Maru
April 22, 2024
1.
What would you advise Jaffer regarding the performance of the new data science algorithm? Ans: Utilizing the method below, the completion rate, click-through rate, and conversion rate for
each model were determined based on the two-week result sets of Vungle's existing advertising
model vs the data science algorithm model.
Completion Rate = Completes/ Impressions
Click Through Rate = Clicks/Impressions
Conversion Rate = Installs/Impressions The calculated average of the completion rate, click-through rate, conversation rate, and eRPM
for both models is given in the below table.
Table 1: Average of both models
Test A
Test B
Average Comepletion rate
0.892712518
0.887846759
Avg Click through rate
0.050677423
0.049239625
Avg conversion rate
0.004027151
0.003538106
Avg eRPM
3.3471
3.459
The campaign aims to raise the eRPM and conversion rate. The average conversion rate, a
crucial metric for gauging the model's effectiveness, indicates that Test-A, the company's
current promotional approach, is doing marginally better when looking at the average table.
Test-A metrics for click-through rate and completion rate perform better than Test-B (data
science advertisement model). But when taking into account Test-B, it performs better than
Test-A in terms of daily average eRPM by $0.131 and $0.119.
Jaffer should run the campaign for a little while longer to see if both models' findings are
consistent, based on my analysis of the aforementioned model and the result set. Jaffer should also attempt to reroute more consumers to Test-B in order to comprehend the
increase in the eRPM and conversation rate, as we are now sending 1 out of every 16 clients
there. Redirecting more customers to Test-B could result in a greater discrepancy between
performance compared to Test-A.
Jaffer can make a decision based on the aforementioned recommendation for the data science model (Test-B), taking into account the difference in eRPM between the model and the current advertising model, as well as the cost of daily maintenance and updates.
It is wise to approve the data science model based on the cost of updating it every day, as well as the eRPM and conversation rate if the ROI is more significant. If not, it is best to stick with the current model and try to enhance it by utilizing machine learning techniques to update it using historical user input and data sets, which reduces the cost of updating the model every day.
Your preview ends here
Eager to read complete document? Join bartleby learn and gain access to the full version
- Access to all documents
- Unlimited textbook solutions
- 24/7 expert homework help
Related Questions
Problem 3-4 (Static)
An electrical contractor's records during the last five weeks indicate the number of job requests:
2 3
22 18
Week:
Requests:
1
20
4
21
Click here for the Excel Data File
Predict the number of requests for week 6 using each of these methods:
a. Naive.
5
22
Answer is complete but not entirely correct.
Number of
requests
24 X
requests
b. A four-period moving average. (Round your answer to 2 decimal places.).
Answer is complete but not entirely correct.
Number of
23.25
c. Exponential smoothing with a = 0.30. Use 20 for week 2 forecast. (Round your intermediate calculations and fina
mal nisenel
arrow_forward
Required information
Camp Rainbow offers overnight summer camp programs for children ages 10–14 every summer during June and
July. Each camp session is one week and can accommodate up to 200 children. The camp is not coed, so boys
attend during the odd-numbered weeks and girls attend during the even-numbered weeks. While at the camp,
participants make crafts, participate in various sports, help care for the camp's resident animals, have cookouts and
hayrides, and help assemble toys for local underprivileged children.
The camp provides all food as well as materials for all craft classes and the toys to be assembled. One cabin can
accommodate up to 10 children, and one camp counselor is assigned to each cabin. Three camp managers are on-
site regardless of the number of campers enrolled.
Following is the cost information for Camp Rainbow's operations last summer:
Number of
Cost to Run
Campers
172
Week
Camp
$13,810
9,350
11,360
14,380
14,000
12,440
10,180
8,440
112
3
148
4
184
5
174
152…
arrow_forward
Question 4 (1 point)
As buyers are increasingly facing information overload, salespeople should ensure information is streamlined and
personalized.
OTrue
OFalse
Question 5 (1 point)
Blogs, white papers, webinars, and presentations should be proyided to targeted customers.
OTrue
OFalse
Question 6 (1 point)
dal fne avaluating solution alternatives uses weighted averages.
arrow_forward
22 my.post.edu/CMCPort X Bb Content
← → C
X Bb Take Test: Chapter 6 X
•
post.blackboard.com/webapps/assessment/take/launch.jsp?course_assessment_id=_290684_1&course_id=_123680_1&content_id=8
Question Completion Status:
QUESTION 8
Probable cause is required for a police officer to conduct a stop and frisk.
O True
O False
QUESTION 7
A law enforcement officer needs reasonable suspicion or probable cause that a crime has taken place when using the "knock and talk" strategy.
O True
O False
QUESTION 9
Which Supreme Court case established the legal parameters of stop and frisk?
O Weeks v Ohio
O Mapp v Ohio
Terry v Ohio
O Carroll v. United States
BO'F
Clear
2
Click Save and Submit to save and submit. Click Save All Answers to save all answers.
HH
Q Search
Academic Writer - Ho X
5
Wordtune Editor
X
G
arrow_forward
Required information
Problem 9-2
[The following information applies to the questions displayed below.]
An air-conditioning repair department manager has compiled data on the primary reason for 41 service calls for the previous week, as shown in the table.
JobNumber
Problem/Customer Type
JobNumber
Problem/Customer Type
JobNumber
Problem/Customer Type
301
O/C
315
W/R
329
N/C
302
N/C
316
F/R
330
O/R
303
F/R
317
F/R
331
N/R
304
O/R
318
N/R
332
W/C
305
F/R
319
F/R
333
W/C
306
N/R
320
N/R
334
N/R
307
N/C
321
O/C
335
F/C
308
O/R
322
W/C
336
W/C
309
N/R
323
F/R
337
F/C
310
N/C
324
O/C
338
N/R
311
O/C
325
W/C
339
N/R
312
O/R
326
N/C
340
N/C
313
N/R
327
O/R
341
N/R
314
O/R
328
N/R
Key:
Problem type:
Customer type:
N
= Noisy
C
= Commercial customer
F
= Equipment failure
R
= Residential customer
W
= Runs warm
O
= Odor
Problem 9-2 Part a
a.
Using the data, prepare a check sheet for the problem…
arrow_forward
A service provided delivering packages to a locker for customers to pick up at their convenience is an example of:
Question content area bottom
Part 1
A.
hybrid office.
B.
passive contact.
C.
active contact.
D.
front office.
arrow_forward
Required:
1. Identify the key problems and issues?
2. Proposed realistic solutions or changes (3 or more)
3. Recommend specific strategy for accomplishing the proposed solutions.
arrow_forward
Calculate:
a. Expected value of perfect information
b. Minimax regret value
arrow_forward
Are my formulas correct and did I get the correct answer?
Question: Sunblessed Juice Company sells bags of oranges and cartons of orange juice. Sunblessed grades oranges on a scale of 1 (poor) to 10 (excellent). At present, Sunblessed has 220,000 pounds of grade 6 oranges and 150,000 pounds of grade 9 oranges on hand. The aver- age quality of oranges sold in bags must be at least 7, and the average quality of the oranges used to produce orange juice must be at least 8. Each pound of oranges that is used for juice yields a revenue of $2.25 and in- curs a variable cost (consisting of labor costs, variable overhead costs, inventory costs, and so on) of $1.35. Each pound of oranges sold in bags yields a revenue of $2.00 and incurs a variable cost of $1.20.
a. Determine how Sunblessed can maximize its profit.
arrow_forward
Question 5 In the context of account classification, which of the following is a benefit of single-factor analysis? O It employs a matrix system. O It is used for goal setting. It requires no data manipulation. O It provides equal weightage to all categories of accounts. O It uses statistical analysis.
arrow_forward
Required information
[The following information applies to the questions displayed below.]
The Air Force Museum Foundation has commissioned the purchase of 16 Four F Sixes, pre-World War II aircraft. They will
be built completely from scratch to the exact specifications used for the originals. As further authentication, the aircraft will
be made using the technology and manufacturing processes available when the originals were built. Each of the 16
airplanes will be flown to Air Force and aviation museums throughout the country for exhibition. Aviation enthusiasts can
also visit the production facility to see exactly how such aircraft were built in 1938.
Soren Industries wants to bid on the aircraft contract and asked for and received certain cost information about the Four F
Sixes from the Air Force. The information includes some of the old cost data from the builders of the original aircraft. The
available information is for the total accumulated time as the 1st, 8th, and 32nd…
arrow_forward
4. (Scenario Based Question) Your response to Dolly's Phone Call & "Hello love, my
name is Dolly Parsons and I am having problems looking at my account details on this
Monzo button app thing on my mobile telephone handset. I really need access to my
pension so I can go shopping for groceries. Gosh I really miss the days of visiting a
bank and speaking to a person and drawing out my money. (**anxious**) Please help
me, I have been trying for 30 mins and I can't open it up and the shops will be closing
soon. My grandson has set me up an email address on my new computer, which I
have written down - dollyparson45@gmail.co.uk Please help me!"
(Write your response to the customer as if you were a COp at Monzo who had just
picked up a call from Dolly, for example "Hi Dolly...")
See all of the information you need to write your answer to this question here
arrow_forward
Problem 7-09 (Algorithmic)
Hawkins Manufacturing Company produces connecting rods for 4- and 6-cylinder automobile engines using the same production line. The cost required to set up the production line to produce the 4-cylinder connecting rods is $1,400, and the cost required to set up the production line for
the 6-cylinder connecting rods is $3,100. Manufacturing costs are $15 for each 4-cylinder connecting rod and $18 for each 6-cylinder connecting rod. Hawkins makes a decision at the end of each week as to which product will be manufactured the following week. If a production
changeover is necessary from one week to the next, the weekend is used to reconfigure the production line. Once the line has been set up, the weekly production capacities are 6,500 6-cylinder connecting rods and 8,200 4-cylinder connecting rods.
Let
X4 = the number of 4-cylinder connecting rods produced next week
X6 = the number of 6-cylinder connecting rods produced next week
S4= 1 if the production line is…
arrow_forward
Saved
Required information
[The following information applies to the questions displayed below.]
Raner, Harris & Chan is a consulting firm that specializes in information systems for medical and dental clinics. The firm has
two offices-one in Chicago and one in Minneapolis. The firm classifies the direct costs of consulting jobs as variable
costs. A contribution format segmented income statement for the company's most recent year is given:
Office
Sales
Variable expenses
Contribution margin
Traceable fixed expenses
Total Company
$ 513,000
256,500
256, 500
143,640
Chicago
$ 171,000
51,300
119,700
88,920
$ 30,780
Minneapolis
$ 342,000
100.00%
50.00%
50.00%
28.00%
100.00%
30.00%
70.00%
52.00%
100.00%
205, 200
136,800
54,720
$ 82,080
60.00%
40.00%
16.00%
Office segment margin
112,860
22.00%
18.00%
24.00%
Common fixed expenses not traceable to
offices
71,820
14.00%
Net operating income
$ 41,040
8.00%
Required:
1-a. Compute the companywide break-even point in dollar sales.
1-b. Compute the…
arrow_forward
Problem 1 Forecasting
• All analysis and calculations and report must be done in a single (ONE) Excel file.• Put your name at the top of the worksheet.• Make Excel do all of the calculations. (Instructor must be able to see your cell-reference formulas.)• Include report/answers below the forecasting calculations. o Make sure answers are clear, complete and easy to find. o Your report must include: a. Presentation of forecasts b. Explanation of why you chose each of the methods
1. Tom Simpson, Director of the Chamber of Commerce for Exeter township is investigating the past ten years oftourist visits to the area. The following data has been gathered on number of tourists who signed into the localinformation center.
Year Number of tourists1 7002 2483 6334 4585 14106 15887 16298 13019 145510 1989
Tom is interested in implementing a forecasting system and is…
arrow_forward
Identify the Information System that would generate the type of report shown below.
DOEJOE INC.
STATEMENT OF OWNER'S EQUITY
FOR MONTH OF AUGUST 21, 2022
JOHN DOE, CAPITAL, AUGUST 31, 2022
INVESTMENT DURING THE PERIOD
NET INCOME FOR PERIOD
SUBTOTAL
WITHDRAWLS DURING THE PERIOD
NET LOSSES FOR PERIOD
JOHN DOE, CAPITAL, AUGUST 31, 2022
O a. Management Information System
O b. Decision support system
O c. Business Intelligence Dashboard
Od. Transaction processing system
4,000.00
1,000.00
2,000.00
$ 7,000.00
2,000.00
$5,000.00
arrow_forward
On November 30, 20A, ABC company received its bank statement. However, the closing balance of the account was unreadable. Attempts to contact the bank after hours did not secure the desired info.
The following data are available in preparing a bank reconciliation:
October 31 book balance 146,000
Note collected by bank 10,000
Interest earned in note 1,000
NSF check of customer 13,000
Bank service charge on NSF check 200
Other bank service charges 300
Outstanding checks 20,200
Deposit of October 31 placed in night depository 8,500
Check issued by LMN company charged to ABC company 2,000
What is the cash balanc per bank statement?
arrow_forward
Please use the proper methodology when solving, thank you.
arrow_forward
Ten weeks of data on the Commodity Futures Index are 7.36, 7.41, 7.56, 7.57, 7.61, 7.53, 7.53, 7.71, 7.63, and 7.56.
(a) Construct a time series plot.
1
2
3
4
What type of pattern exists in the data?
Week
5
The data appear to follow a horizontal pattern.
O The data appear to follow a cyclical pattern.
O The data appear to follow a trend pattern.
O The data appear to follow a seasonal pattern.
6
8.5-
8.3
8.1
7.9+
7
7.7
7.5-
8
7.3
7.1
6.9
6.7
6.5 +
01 23 4567 7 8 9 10 11
Week
9
8.5-
8.3-
10
8.1
7.9
7.7+
7.5-
7.3
7.1
6.9
6.7
6.5
0 1 2
Time Series
Value
7.36
7.41
7.56
(b) Use trial and error to find a value of the exponential smoothing coefficient a that results in a relatively small MSE. (Round
your answers to two decimal places.)
7.57
7.61
7.53
3 4
7.53
7.71
5 67 7 8 9 10 11
Week
7.63
7.56
a = 0.1
Forecast
α = 0.3
Forecast
J
C
O
Index
DIC
a = 0.8
Forecast
8.5
8.3-
8.1
7.9
7.7
7.5-
7.3
7.1
6.9
6.7
6.5
0
8.5 T
8.3-
8.1
7.9-
7.7-
7.5
7.3
7.1
6.9-
6.7-
6.5
1
2 3 4 5 678
Week
+
0 1 2 3 4
8 9…
arrow_forward
markting question....when is the customer said to be satisfied ?
arrow_forward
SEE MORE QUESTIONS
Recommended textbooks for you
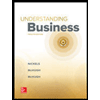
Understanding Business
Management
ISBN:9781259929434
Author:William Nickels
Publisher:McGraw-Hill Education
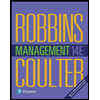
Management (14th Edition)
Management
ISBN:9780134527604
Author:Stephen P. Robbins, Mary A. Coulter
Publisher:PEARSON
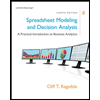
Spreadsheet Modeling & Decision Analysis: A Pract...
Management
ISBN:9781305947412
Author:Cliff Ragsdale
Publisher:Cengage Learning
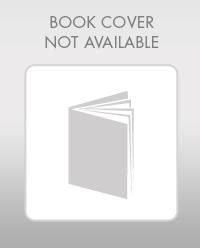
Management Information Systems: Managing The Digi...
Management
ISBN:9780135191798
Author:Kenneth C. Laudon, Jane P. Laudon
Publisher:PEARSON
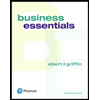
Business Essentials (12th Edition) (What's New in...
Management
ISBN:9780134728391
Author:Ronald J. Ebert, Ricky W. Griffin
Publisher:PEARSON
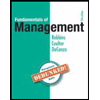
Fundamentals of Management (10th Edition)
Management
ISBN:9780134237473
Author:Stephen P. Robbins, Mary A. Coulter, David A. De Cenzo
Publisher:PEARSON
Related Questions
- Problem 3-4 (Static) An electrical contractor's records during the last five weeks indicate the number of job requests: 2 3 22 18 Week: Requests: 1 20 4 21 Click here for the Excel Data File Predict the number of requests for week 6 using each of these methods: a. Naive. 5 22 Answer is complete but not entirely correct. Number of requests 24 X requests b. A four-period moving average. (Round your answer to 2 decimal places.). Answer is complete but not entirely correct. Number of 23.25 c. Exponential smoothing with a = 0.30. Use 20 for week 2 forecast. (Round your intermediate calculations and fina mal nisenelarrow_forwardRequired information Camp Rainbow offers overnight summer camp programs for children ages 10–14 every summer during June and July. Each camp session is one week and can accommodate up to 200 children. The camp is not coed, so boys attend during the odd-numbered weeks and girls attend during the even-numbered weeks. While at the camp, participants make crafts, participate in various sports, help care for the camp's resident animals, have cookouts and hayrides, and help assemble toys for local underprivileged children. The camp provides all food as well as materials for all craft classes and the toys to be assembled. One cabin can accommodate up to 10 children, and one camp counselor is assigned to each cabin. Three camp managers are on- site regardless of the number of campers enrolled. Following is the cost information for Camp Rainbow's operations last summer: Number of Cost to Run Campers 172 Week Camp $13,810 9,350 11,360 14,380 14,000 12,440 10,180 8,440 112 3 148 4 184 5 174 152…arrow_forwardQuestion 4 (1 point) As buyers are increasingly facing information overload, salespeople should ensure information is streamlined and personalized. OTrue OFalse Question 5 (1 point) Blogs, white papers, webinars, and presentations should be proyided to targeted customers. OTrue OFalse Question 6 (1 point) dal fne avaluating solution alternatives uses weighted averages.arrow_forward
- 22 my.post.edu/CMCPort X Bb Content ← → C X Bb Take Test: Chapter 6 X • post.blackboard.com/webapps/assessment/take/launch.jsp?course_assessment_id=_290684_1&course_id=_123680_1&content_id=8 Question Completion Status: QUESTION 8 Probable cause is required for a police officer to conduct a stop and frisk. O True O False QUESTION 7 A law enforcement officer needs reasonable suspicion or probable cause that a crime has taken place when using the "knock and talk" strategy. O True O False QUESTION 9 Which Supreme Court case established the legal parameters of stop and frisk? O Weeks v Ohio O Mapp v Ohio Terry v Ohio O Carroll v. United States BO'F Clear 2 Click Save and Submit to save and submit. Click Save All Answers to save all answers. HH Q Search Academic Writer - Ho X 5 Wordtune Editor X Garrow_forwardRequired information Problem 9-2 [The following information applies to the questions displayed below.] An air-conditioning repair department manager has compiled data on the primary reason for 41 service calls for the previous week, as shown in the table. JobNumber Problem/Customer Type JobNumber Problem/Customer Type JobNumber Problem/Customer Type 301 O/C 315 W/R 329 N/C 302 N/C 316 F/R 330 O/R 303 F/R 317 F/R 331 N/R 304 O/R 318 N/R 332 W/C 305 F/R 319 F/R 333 W/C 306 N/R 320 N/R 334 N/R 307 N/C 321 O/C 335 F/C 308 O/R 322 W/C 336 W/C 309 N/R 323 F/R 337 F/C 310 N/C 324 O/C 338 N/R 311 O/C 325 W/C 339 N/R 312 O/R 326 N/C 340 N/C 313 N/R 327 O/R 341 N/R 314 O/R 328 N/R Key: Problem type: Customer type: N = Noisy C = Commercial customer F = Equipment failure R = Residential customer W = Runs warm O = Odor Problem 9-2 Part a a. Using the data, prepare a check sheet for the problem…arrow_forwardA service provided delivering packages to a locker for customers to pick up at their convenience is an example of: Question content area bottom Part 1 A. hybrid office. B. passive contact. C. active contact. D. front office.arrow_forward
- Required: 1. Identify the key problems and issues? 2. Proposed realistic solutions or changes (3 or more) 3. Recommend specific strategy for accomplishing the proposed solutions.arrow_forwardCalculate: a. Expected value of perfect information b. Minimax regret valuearrow_forwardAre my formulas correct and did I get the correct answer? Question: Sunblessed Juice Company sells bags of oranges and cartons of orange juice. Sunblessed grades oranges on a scale of 1 (poor) to 10 (excellent). At present, Sunblessed has 220,000 pounds of grade 6 oranges and 150,000 pounds of grade 9 oranges on hand. The aver- age quality of oranges sold in bags must be at least 7, and the average quality of the oranges used to produce orange juice must be at least 8. Each pound of oranges that is used for juice yields a revenue of $2.25 and in- curs a variable cost (consisting of labor costs, variable overhead costs, inventory costs, and so on) of $1.35. Each pound of oranges sold in bags yields a revenue of $2.00 and incurs a variable cost of $1.20. a. Determine how Sunblessed can maximize its profit.arrow_forward
- Question 5 In the context of account classification, which of the following is a benefit of single-factor analysis? O It employs a matrix system. O It is used for goal setting. It requires no data manipulation. O It provides equal weightage to all categories of accounts. O It uses statistical analysis.arrow_forwardRequired information [The following information applies to the questions displayed below.] The Air Force Museum Foundation has commissioned the purchase of 16 Four F Sixes, pre-World War II aircraft. They will be built completely from scratch to the exact specifications used for the originals. As further authentication, the aircraft will be made using the technology and manufacturing processes available when the originals were built. Each of the 16 airplanes will be flown to Air Force and aviation museums throughout the country for exhibition. Aviation enthusiasts can also visit the production facility to see exactly how such aircraft were built in 1938. Soren Industries wants to bid on the aircraft contract and asked for and received certain cost information about the Four F Sixes from the Air Force. The information includes some of the old cost data from the builders of the original aircraft. The available information is for the total accumulated time as the 1st, 8th, and 32nd…arrow_forward4. (Scenario Based Question) Your response to Dolly's Phone Call & "Hello love, my name is Dolly Parsons and I am having problems looking at my account details on this Monzo button app thing on my mobile telephone handset. I really need access to my pension so I can go shopping for groceries. Gosh I really miss the days of visiting a bank and speaking to a person and drawing out my money. (**anxious**) Please help me, I have been trying for 30 mins and I can't open it up and the shops will be closing soon. My grandson has set me up an email address on my new computer, which I have written down - dollyparson45@gmail.co.uk Please help me!" (Write your response to the customer as if you were a COp at Monzo who had just picked up a call from Dolly, for example "Hi Dolly...") See all of the information you need to write your answer to this question herearrow_forward
arrow_back_ios
SEE MORE QUESTIONS
arrow_forward_ios
Recommended textbooks for you
- Understanding BusinessManagementISBN:9781259929434Author:William NickelsPublisher:McGraw-Hill EducationManagement (14th Edition)ManagementISBN:9780134527604Author:Stephen P. Robbins, Mary A. CoulterPublisher:PEARSONSpreadsheet Modeling & Decision Analysis: A Pract...ManagementISBN:9781305947412Author:Cliff RagsdalePublisher:Cengage Learning
- Management Information Systems: Managing The Digi...ManagementISBN:9780135191798Author:Kenneth C. Laudon, Jane P. LaudonPublisher:PEARSONBusiness Essentials (12th Edition) (What's New in...ManagementISBN:9780134728391Author:Ronald J. Ebert, Ricky W. GriffinPublisher:PEARSONFundamentals of Management (10th Edition)ManagementISBN:9780134237473Author:Stephen P. Robbins, Mary A. Coulter, David A. De CenzoPublisher:PEARSON
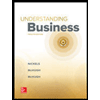
Understanding Business
Management
ISBN:9781259929434
Author:William Nickels
Publisher:McGraw-Hill Education
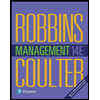
Management (14th Edition)
Management
ISBN:9780134527604
Author:Stephen P. Robbins, Mary A. Coulter
Publisher:PEARSON
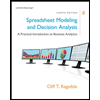
Spreadsheet Modeling & Decision Analysis: A Pract...
Management
ISBN:9781305947412
Author:Cliff Ragsdale
Publisher:Cengage Learning
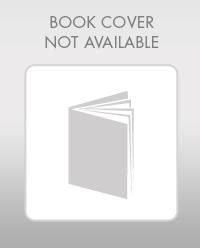
Management Information Systems: Managing The Digi...
Management
ISBN:9780135191798
Author:Kenneth C. Laudon, Jane P. Laudon
Publisher:PEARSON
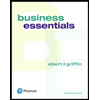
Business Essentials (12th Edition) (What's New in...
Management
ISBN:9780134728391
Author:Ronald J. Ebert, Ricky W. Griffin
Publisher:PEARSON
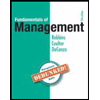
Fundamentals of Management (10th Edition)
Management
ISBN:9780134237473
Author:Stephen P. Robbins, Mary A. Coulter, David A. De Cenzo
Publisher:PEARSON